The ability of AI to mitigate risk remains one of the most critical areas of development for financial institutions even though the rich world of chatbots is associated the most with developments of artificial intelligence. Although the average support center call is estimated to cost $4.00 in the US, adding up to a significant expense if a support center receives hundreds or thousands of calls per day, it does not compare to the cost and damage of various forms of fraud to banks, consumers, and merchants. E.g., the Ingenico Group estimates that merchants lose on average 1.5% of their annual revenue to fraud attacks. This 1.5% represents product and service losses, chargeback fees, and potential scheme programs. Meanwhile, identity theft, fraud costs consumers more than $16 billion.
AI-powered risk management for financial institutions is embodied in advanced fraud prevention and AML solutions, as well as more accurate customer assessment. However, AI has a more defining and more rarely discussed impact – stress testing result submissions and the adjustment of capital requirements for institutions.
Stress testing submissions are vitally crucial for regulatory compliance. At the end of 2017, Ayasdi shared the results of its work with Citi, one of the world’s largest and most complex financial institutions, operating in 98 countries, facilitating more than $4 trillion in flows each day. They hold over $950 billion in deposits and have over $620 billion in loans across their institutional and consumer businesses.
Explaining the background of the case, the company shares: “The 2008-09 financial collapse led to a Federal Reserve directive that banks with consolidated assets over $50 billion have additional risk assessment frameworks and budgetary oversight in place. To assess a bank’s financial foundation, the Federal Reserve oversees several scenarios (company-run stress tests). Referred to as the Comprehensive Capital Analysis and Review (CCAR) process, these tests are meant to measure the sources and use of capital under baseline as well as stressed economic and financial conditions to ensure capital adequacy in all market environments.”
As Ayasdi reports, Citi consistently struggled to pass its annual stress test, failing two of the first three stress tests. As a result, the bank needed a way to rapidly create accurate, defensible models that would prove to the Federal Reserve that they could adequately forecast revenues and the capital reserve required to absorb losses under stressed economic conditions. As a result, the firm could not confidently defend the models they included in the filings they presented to the Federal Reserve. And to address the issue, Citi chose Ayasdi to supplement its capital planning process.
Regulatory bodies are equally interested in adopting advanced technologies to tackle the issue. For example, the Financial Stability Board (FSB) recently published a report sharing that some regulators are using AI for fraud and AML/CFT detection.
“The Australian Securities and Investments Commission (ASIC) has been exploring the quality of results and potential use of NLP technology to identify and extract entities of interest from evidentiary documents. ASIC is using NLP and other technology to visualize and explore the extracted entities and their relationships. For example, to fight criminal activities carried out through the banking system (such as money laundering), BdI collects detailed information on bank transfers and correlates this information with information from newspaper articles. The correlation involves both structured and unstructured data for file sizes of more than 50 gigabytes.”
FSB also reports that ASIC has also used machine learning software to identify misleading marketing in a particular sub-sector, such as unlicensed accountants in the provision of financial advice.
FSB also shares an example of the Monetary Authority of Singapore (MAS) exploring the use of AI and ML in analyzing suspicious transactions to identify those transactions that warrant further attention, allowing supervisors to focus their resources on higher-risk transactions.
“Investigating suspicious transactions is time-consuming and often suffers from a high rate of false positives due to defensive filings by regulated entities. Machine learning is being used to identify complex patterns and highlight the suspicious transactions that are potentially more serious and warrant closer investigation. Coupled with machine learning methods to analyze the granular data from transactions, client profiles, and a variety of unstructured data, machine learning is being explored to uncover non-linear relationships among different attributes and entities and to detect potentially complicated behavior patterns of money laundering and the financing of terrorism not directly observable through suspicious transactions filings from individual entities.”
Another regulatory body, the US Securities and Exchange Commission (SEC), for example, leverages big data to develop text analytics and machine learning algorithms to detect possible fraud and misconduct.
“The SEC staff uses machine learning to identify patterns in the text of SEC filings. These patterns can be compared to past examination outcomes with supervised learning to find risks in investment manager filings. The SEC staff notes that these techniques are five times better than random at finding language that merits a referral to enforcement.”
Unsupervised learning algorithms are used by SEC to identify unique or outlier reporting behaviors – including both topic modeling and tonality analysis.
Risk management is of the highest priority for financial institutions and regulators in the financial sector because of far-reaching consequences. The ripple of massive fraud cases touches every party in the ecosystem – the customer, the institution, and businesses. In response to the need of banks such as Citi and regulators, technology companies offer answers with a great promise. For example, at the end of 2017, Intel launched the Intel Saffron AML Advisor, aimed at detecting financial crime through an AI solution utilizing associative memory. Intel Saffron was the first associative memory AI solution specifically tailored to the needs of financial services institutions and optimized on Intel Xeon Scalable processors.
An extensive community of tech startups also explores the field, sharing significant achievements in trials and tests.
Bloomberg reports that as financial services firms continue to improve their compliance and risk management processes and systems, many are putting artificial intelligence to work to augment their current processes.
“The ability of machine learning models to analyze large amounts of data both financial and non-financial – with more granularity and deeper analysis – can improve analytical capabilities in risk management and compliance, helping analysts make more informed decisions at a securities level and across a broad-based, multi-asset portfolio.”
To learn about Prove’s identity solutions and how to accelerate revenue while mitigating fraud, schedule a demo today.

Keep reading
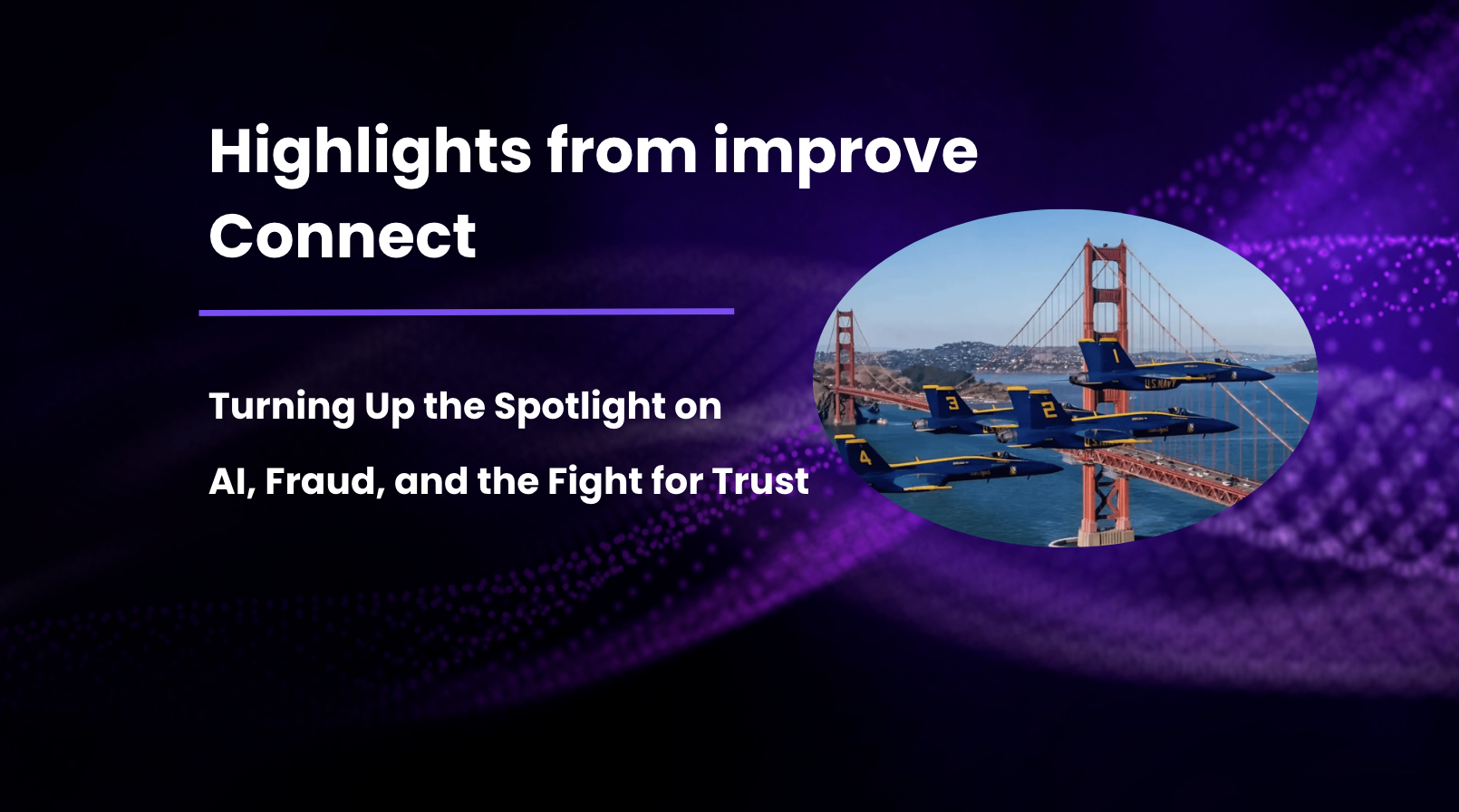
Industry leaders gathered at Prove's Improve Connect summit to discuss balancing frictionless digital experiences with the threat of AI-powered fraud. Experts from companies like Coinbase, Bluevine, and Google shared insights on navigating the challenges and opportunities of emerging technologies.

Digital identity theft is the new frontier of crime, where criminals steal our online "keys" to unlock financial accounts, social media, and even medical records, causing widespread damage and eroding trust in the digital world. Companies inadvertently contribute to this problem by failing to adequately protect user data with secure software development practices.

As organizations in the UK prioritize business growth through online transactions, establishing trust & safety with users is rapidly becoming the critical element that offers a competitive advantage.