Artificial intelligence and machine learning saw a significant spike of attention in the past few years – whether it’s through partnerships, acquisitions, or in-house developments. The largest financial institutions in the US have been involved in one way or another in bringing artificial intelligence into operations and customer-facing functions.
A recent study of 34 major banks across several geographies (US, EU, Singapore, Africa, Australia, India) found that 27 out of these 34 banks have implemented AI in their front-office functions in the form of a chatbot, virtual assistant, and digital advisor. Some of the most prominent banks in this space across regions are Bank of America, OCBC, ABN Amro, YES BANK, etc. While front-office applications have certainly seen a higher intensity, scope, and adoption, the AI strategy in the US banking industry, in reality, is far more diverse.
As for the US, all major banks have been found to be experimenting with artificial intelligence in one of the four sets of applications: front-office uses; back-office uses; trading and portfolio management in financial markets; and uses of AI and machine learning by financial institutions for regulatory compliance (RegTech) or by public authorities for supervision (SupTech).
Let’s look at particular use cases of artificial intelligence that are currently being explored by the largest financial institutions in the US.
Automation
RPA is expected to have a $6.7 trillion global economic impact, a plan that will result in a 40-45% growth of global spending on technology. In addition, the estimated global market potential of RPA stands at $8.75 billion by 2024. Automation is one of the more explored areas of AI/ML application.
Barclays Bank, for example, has implemented RPA across various processes which include but are not limited to – fraud detection, risk monitoring, account receivables processing, and loan application. With the implementation of RPA enhancing productivity, Barclays was able to save over £175 million annually in bad debt provisioning.
Another large financial institution has focused on back-office automation through RPA. The bank reported that the implementation of RPA has led to the following results: 100% accuracy in account-closure validations across five systems, 88% improvement in processing time, 66% improvement in trade entry turnaround time, ¼-second robotic reconciliation of a failed trade vs. 5-10 minutes by a human. The activity of its fund transfer bots alone is responsible for $300,000 in annual savings.
One of the latest and probably most vivid indicators of transformative processes in banking is Goldman Sachs, which brings significant automation into areas of trading like currencies and futures using complex trading algorithms, some with machine learning capabilities.
Citibank invested in Ayasdi and uses its technology in several use cases, including automating data-to-value processes and reducing time to insights.
Compliance, cybersecurity
One of the largest financial institutions in the US has introduced its own intelligent platform designed to analyze legal documents and extract important data points and clauses. The use of AI reduced the review of 12,000 annual commercial agreements from hundreds of thousands of man-hours to a few seconds. The program developed by the bank does the job of interpreting commercial-loan agreements that, until the project went online in June 2016, consumed 360,000 hours of work each year by lawyers and loan officers. The software reviews documents in seconds and is not prone to errors.
The same firm set up technology hubs for teams specializing in big data, robotics, and cloud infrastructure to find new sources of revenue while reducing expenses and risks. The system already is helping the bank automate some coding activities and making its 20,000 developers more productive, saving money.
Cybersecurity, closely tied to standards of the regulatory framework in the market, is no longer a financial liability. Rather, it’s a competitive advantage of the modern day. To bring the capabilities if intelligent machines into risk management, TD Bank, for example, acquired an AI startup, Layer6. Layer 6 team has built an AI prediction engine that can ingest a variety of data types – customer profiles, transaction histories, phone calls, images (photos and documents), and video – and be trained to make predictions, such as what the next best action for a customer is. The more important part is that it can also be applied to detecting fraud, scoping out cybersecurity threats and underwriting loans.
Being one of the most forward-thinking institutions, Goldman Sachs, for example, has strong ties (as a customer and as an investor) with an AI software provider, Digital Reasoning, whose solution GS uses to track traders.
The same startup has also launched a program with NASDAQ to use its AI technology to track trading data, communications, emails, chats, and even voice data to ferret out misconduct across the entire electronic stock exchange.
One of the most interesting startups on the radar of financial institutions allows to identify and eradicate fraud in all avenues of commerce, including online and in-person banking, in real time. Through its continuous and rapid evaluation of large amounts of data, the company can conduct large-scale analyses, and fraudulent or questionable activity is identified and the customer is rapidly alerted. The service also assists payment providers and retailers in monitoring and protecting financial activity in connection with their companies.
Lloyds Banking Group has partnered with AI startup Pindrop to use its machine learning technology to detect fraudulent phone calls. Pindrop can identify 147 different features of a voice from a phone call or even a Skype call, which can help a person identify information such as the location that a caller is in creating an audio fingerprint. Lloyds Banking Group will introduce the software across the Lloyds Bank, Halifax, and Bank of Scotland brands. Lloyds said the partnership with Pindrop will help it cut down call times and protect customers.
Investment banking, trading
Investment banking and trading have been somewhat controversial in yielding results when AI was brought into space, although continuously hold a lot of promise. For example, hedge funds that use artificial intelligence and machine learning in their trading process posted the worst month on record in February 2018, according to a Eurekahedge index that’s tracked the industry from 2011. While computerized programs are feared for their potential to render human traders obsolete, the AI quants lagged behind their discretionary counterparNonetheless, investment banking and tradingess, are some of the most important and interesting areas with high stakes. The very same institutions that are exploring the capabilities of AI in automation and regulatory functions have also been experimenting with using ML in investment banking. One of the institutions in the US last year launched a predictive recommendation engine to identify clients that should issue or sell equity. After some initial success, the bank is expanding this into other areas such as debt capital markets.
The same bank has also partnered with a tech company to deploy a data analytics and ML program in the bank’s fixed-income sales and trading operations. The solution is supposed to compile data from all desks and orders to give salespeople and traders a clearer picture in real time and help them anticipate market moves.
Wells Fargo analysts, for example, built a robot called AIERA (artificially intelligent equity research analyst), which is now tracking 13 stocks.
“AIERA’s primary purpose is to track stocks and formulate a daily, weekly and overall view on whether the stocks tracked will go up or down,” said Ken Sena, Managing Director, Global Internet Analyst, Wells Fargo Securities. “View AIERA as enhancing versus replacing.”
The months spent developing the bot helped the team of analysts deepen their understanding of the artificial intelligence and machine learning capabilities used by many internet companies they analyze. While AIERA is not picking stocks in the traditional sense yet, her validity tests continue to indicate above average.
Payments, reconciliation
AI software has been shown to improve companies’ straight-through reconciliation of incoming payments. Aiming at large or complex companies that are seeking to reduce costs, decrease days sales outstanding (DSO), and improve cash forecasting & their end-customer experience, one of the solutions in the market is suited for companies that manage a large volume of payments & e-invoices, where the remittance information is either missing or received separately from the payment. The tool identifies payers and associates’ payments to remittances that are received separately; extracts remittance data from emails, email attachments, electronic data interchange (EDI) and payer web portals; matches e-invoices to open receivables using the enriched remittance data and creates a receivable posting file that the client uploads to their ERP system.
Wealth management
Wealth management has seen the adoption of advanced technologies in the face of robo-advisors. In 2016, assets managed by robo-advisors were estimated to increase by 68% annually and reach ~$2.2 trillion in five years. Other estimations suggested that robo-advisors will be managing $8 trillion globally by 2020.
Morgan Stanley’s wealth management unit is testing the AI/ML upgrade to its next-best-action system from rule-based approaches to suggesting investment options to a system that employs machine learning to match investment possibilities to client preferences and helps its 16,000 financial advisors. The next-best-action system also enables automated alert generation on events such as margin call, low balance, significant increase/decrease in the portfolio, Brexit impact, etc. With the debate on humans vs. robo-advisors finally over, this application empowers FAs to better serve their customers by setting up personalized messages with alerts. Morgan Stanley’s system also sends recommendations based on life events. For example, a client had a child with a certain illness; the system could recommend the best local hospitals, schools, and financial strategies for dealing with the illness. Morgan Stanley plans to release a digital-only version with managed portfolios eventually. It will be offered at a lower cost level to clients who prefer digital-only channels.
Virtual assistants, chatbots
Finally, the one use case that has seen the most significant interest, media coverage, and development is the area of consumer interactions – the front-end, encompassing applications in credit scoring, insurance, and client-facing chatbots. In July 2017, Wells Fargo became the first US bank with a Facebook Messenger chatbot. This virtual assistant communicates with users to provide account information and helps customers reset their passwords.
Another bank – Bank of America – launched an intelligent virtual assistant named Erica, a chatbot leveraging predictive analytics and cognitive messaging to provide financial guidance to over 45 million customers. It will be accessible to clients 24/7 and perform day-to-day transactions, understand their unique financial needs and help them reach their financial goals by providing smart recommendations.
A large NY-based institution is building a voice-controlled artificial intelligence platform to help its IT staff manage enterprise storage. The AI chatbot is expected to be available to thousands of IT employees to help them automate manual storage-related tasks. IT employees will be able to communicate by voice or text with the chatbot, integrated with the enterprise collaboration tool Skype for Business, on a desktop.
“The biggest focus of banks with their AI initiatives is conversational interfaces and virtual assistants, closely followed by process excellence and fraud detection. While a relatively fewer number of banks are looking at AI in compliance and trading functions, there is no shortage of opportunities to be explored in these spaces. As the year 2018 unfolds, we may expect several new acquisitions/partnerships by banks and AI startups. These partnerships will play a major role in defining the perimeter for the evolving AI strategies of banks.” – Diwakar Mandal, MEDICI
To learn about Prove’s identity solutions and how to accelerate revenue while mitigating fraud, schedule a demo today.

Keep reading

Prove’s solutions can help businesses make their online customer experiences faster, easier and more secure.
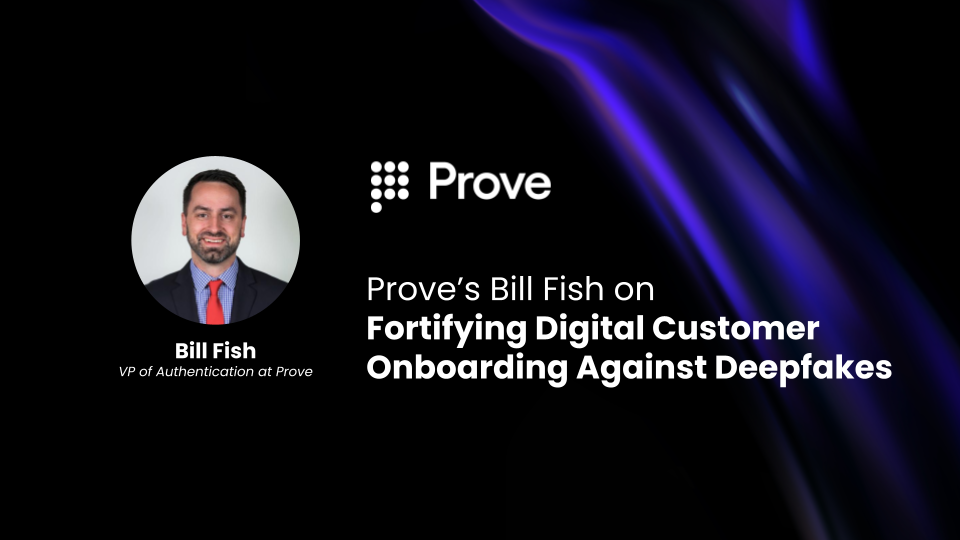
While the rise of deepfake technology is not totally new, its level of sophistication presents new challenges for businesses seeking to deliver frictionless digital onboarding experiences to their customers.

Prove and BetMGM, the sports betting and iGaming leader, have entered into a partnership which will elevate the security standards and user experience for BetMGM customers through the Prove Pre-Fill® identity solution.